Research Progress
However, two major problems are hindering the development of accurate μXRD image screening. One is the lack of labeled industrial samples and the other is the privacy concerns of industrial users of μXRD services.
To address these problems, a research group led by Professor ZHU Yongxin at the Shanghai Advanced Research Institute, Chinese Academy of Sciences unveiled the solutions to improve the screening while protecting data privacy.
The results of the physics law-informed federated learning (FL) based μXRD image screening method are published on IEEE TRANSACTIONS ON INDUSTRIAL INFORMATICS.
Researchers firstly utilized the domain specific physical information to improve the federated learning accuracy. Then, with the consideration of the unbalanced data distributions in realistic world, they adopted a sampling scheme with novel client sampling algorithms. Thirdly, a hybrid training framework was proposed to deal with the unstable communication environment between FL clients and servers in federated learning.
The extensive experiments showed that the accuracy of machine learning models got improved by around 14% to 25% and the data features could be shared among different users or applications while keeping commercially confidential information.
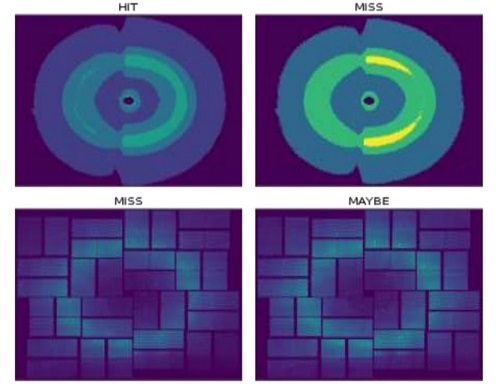
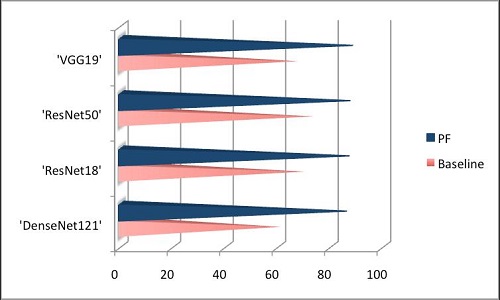
Fig. 2. Improved accuracy in connection with various plug-in models (Image by SARI)